What is a Distributed In-Memory Data Store and How Can it Benefit Your Business?
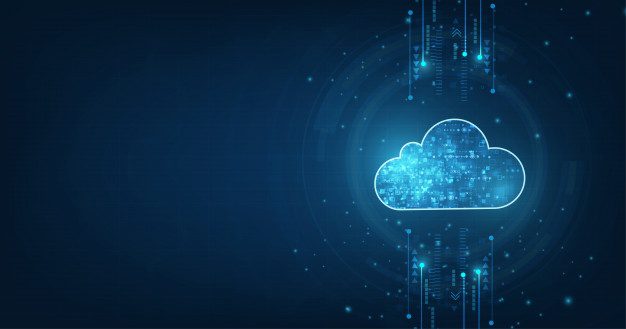
Digital transformation brings attention to technical drawbacks this migration has for enterprise systems. Organizations using legacy data processing systems and enterprises starting from scratch face a complex set of problems. One of those problems is reducing data query latency while processing insights for multiple enterprise systems such as user-end applications and BI tools. Data management engineers continue to design advanced systems like distributed in-memory data stores. What is a distributed in-memory data store, and how can it benefit your business?
What is a distributed in-memory data store?
The advent of distributed systems improved the data processing landscape by a long shot. Enterprise systems took a major leap from using disk-based data processing solutions with major disadvantages in this day and age. A distributed in-memory data grid utilizes multiple nodes to read, write and persist information between front-end enterprise systems and EDWs.
Distributed in-memory data stores are highly effective because they are designed to eliminate the negative effects service failures would have on operational insights. This solution is an extension of regular in-memory databases but utilizes a grid of nodes that process operational data.
Instead of storing the insights on a single in-memory module, data is stored on multiple nodes. Distributed in-memory data stores are very different from disk-based or SSD-powered databases. To read, write and persist data, in-memory databases (IMDBs) use memory instead of fetching and configuring insights directly on disks. The functionality of IMDBs is replicated for higher efficacy, accuracy, and reliability on distributed in-memory data stores.
Distributed in-memory data store architecture
Distributed in-memory data stores have a more complex architecture than regular IMDBs. Since they are distributed, the data store infrastructure is integrated to store data on memory modules like RAM. The entire architecture stack is designed to make operational data processing more efficient and swift than any other solution currently available.
Data management engineers design in-memory data stores using cloud-based SaaS or IaaS vendors with the distributed infrastructure to host this advanced insight processing solution. The data store can be used to complete a larger stack of data processing, especially if the insights have to be persisted to a more permanent storage location.
For example, data engineers or system architects can design a solution entailing a distributed in-memory data store and persisting insights to an EDW. If the datastore is only for short-term reporting and agile Business Intelligence (BI), the architecture can be simpler. Distributed systems are designed similarly to the technology behind crypto trading platforms since they are distributed across several nodes.
Use cases of in-memory data stores
Distributed in-memory data stores can be used for applications requiring microsecond insight processing capabilities. With the data distributed across multiple in-memory modules, it is highly available and can be accessed or written faster than you can blink.
There are countless applications of swift data processing like this. One of the major markets that demand distributed in-memory data stores is real-time online bidding industries. For example, auction websites or other bidding platforms require real-time feedback with minimal chances of latency. Another perfect use case of distributed in-memory data stores is powering gaming leaderboards or competition giveaway scoreboards.
Additionally, this type of insight processing solution has a lot of applications in the fintech industry. Fintech developers can use this distributed system to provide real-time feedback to trading and securities platforms. Market prices can be displayed in real-time to front-end interfaces for end-users, and data is also written back to the operational data store within micro or milliseconds. Know more about Full stack developer course with placement guarantee.
Cost-saving by using this datastore
One major benefit the organizations can derive from using distributed in-memory data stores is reaching cost-saving targets more easily than ever before. This processing solution simplifies processes and removes the use of older technologies that increase the price tag of data management.
Legacy data management systems used disk-based processing solutions that increased the price of storing and processing insights. Not to mention the steep prices of buying and maintaining the infrastructure. Data farms attempted to reduce these costs, but they were still a bit too expensive for high-end enterprise systems.
Fast-forward to today, implementing data processing for complex enterprise system applications has never been easier or cheaper. Since in-memory data stores are available as cloud-based SaaS or IaaS solutions, they are significantly more affordable. In fact, this type of data store can be paid for as a pay-as-you-go service which makes it significantly more affordable than other processing systems.
Distributed IMDB scalability
In addition to distributed in-memory data stores being affordable, they are also highly scalable. Since most cloud-based vendors offer a pay-as-you-go solution package, enterprise system developers can scale their data processing with more freedom. Legacy disk-based processing systems required more infrastructure to scale operations to have larger storage.
Using distributed data store systems simplifies this issue by providing enterprise system developers the latitude to scale operations conveniently. During peak seasons, companies can scale up their distributed in-memory data storage capacity. In other seasons that are less busy, organizations can reduce their storage capacity and processing rate. That is the freedom afforded by cloud-based distributed systems for enterprise system developers.
Migrating to this solution can be both cost-effective and provide easier scalability, especially if the solution is pay-as-you-go. Even with regular solution packages, it is more convenient to scale data processing operations according to the current demand instead of maintaining infrastructure that’s inoperational most of the time.
Reliability and optimum performance
Reliability and performance rating is critical for organizational success in a digitally transforming business world. There is plenty of competition standing by to snatch away dissatisfied customers. This is a possibility when using regular in-memory solutions instead of opting for a distributed system.
If the system crashes and loses data, regular in-memory solutions might not have a backup since the insights are not yet persisted to a permanent storage location. Distributed in-memory data stores offer both reliability and optimum performance since insights can be pulled from the grid.
Therefore, if one node fails, the other will facilitate that transaction or data query. In a nutshell, there is minimal downtime, and the enterprise systems can provide data processing at high bandwidth capacity with significantly reduced latency.